Thank you for visiting nature.com. You are using a browser version with limited support for CSS. To obtain the best experience, we recommend you use a more up to date browser (or turn off compatibility mode in Internet Explorer). In the meantime, to ensure continued support, we are displaying the site without styles and JavaScript.
Scientific Data volume 10, Article number: 342 (2023 ) Cite this article Panasonic Ionizer
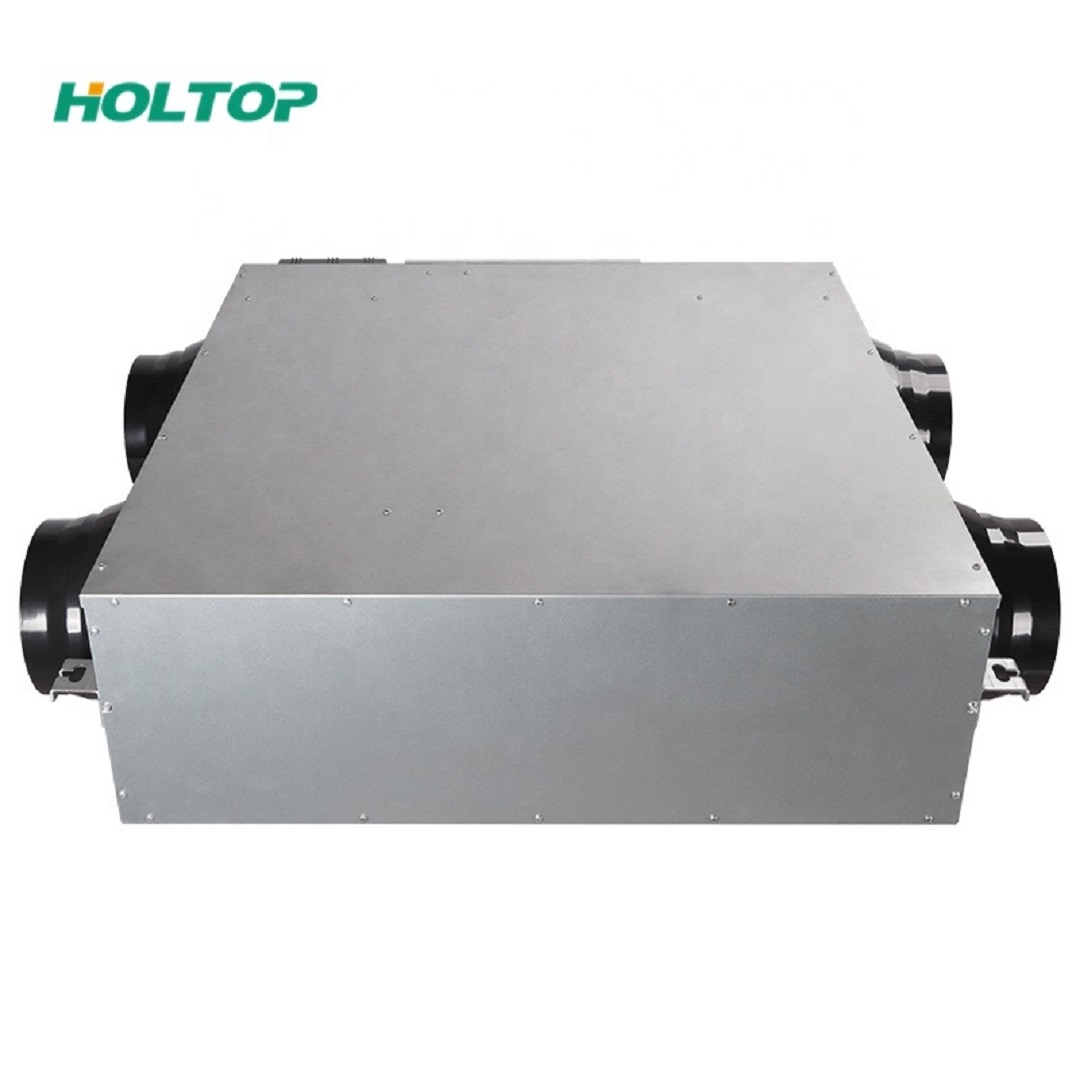
Open data is fueling innovation across many fields. In the domain of building science, datasets that can be used to inform the development of operational applications - for example new control algorithms and performance analysis methods - are extremely difficult to come by. This article summarizes the development and content of the largest known public dataset of building system operations in faulted and fault free states. It covers the most common HVAC systems and configurations in commercial buildings, across a range of climates, fault types, and fault severities. The time series points that are contained in the dataset include measurements that are commonly encountered in existing buildings as well as some that are less typical. Simulation tools, experimental test facilities, and in-situ field operation were used to generate the data. To inform more data-hungry algorithms, most of the simulated data cover a year of operation for each fault-severity combination. The data set is a significant expansion of that first published by the lead authors in 2020.
Jessica Granderson, Guanjing Lin, … Yan Chen
Clayton Miller, Anjukan Kathirgamanathan, … Forrest Meggers
Yeobeom Yoon, Sungkyun Jung, … Anthony Gehl
Fault detection and diagnostics (FDD) is a well-established field of study in building science and building technology applications. This is largely driven by the significant impact of equipment faults and control problems on building energy use and emissions, equipment life, and occupant comfort. Building HVAC systems in particular, afford a rich opportunity space for FDD algorithm development, given the multiplicity of system configurations, complex operations, and availability of monitored data. In addition, the recent push to decarbonize buildings and the electricity sector is increasing the importance of grid-interactive efficient buildings that can reliably provide load-flexibility services to the renewable supplied grid. This is making it even more critical to ensure that building HVAC systems are controllable and fault-free, providing further motivation for FDD technology development and deployment.
In buildings, FDD software tools employ operational data collected from building automation systems, sensors, and meters, to automatically detect equipment and control problems, or degrading performance in an HVAC system, and to diagnose potential root causes1. Using the results from FDD technologies, building operators can efficiently direct maintenance activities to address inefficiencies, or equipment and control malfunctions.
In the past thirty years, a large body of literature has been published documenting the development and application of FDD solutions for buildings. The active research covers a breadth of topics including: (1) the development and validation of hundreds of FDD methods2,3,4; (2) the development of experimental platforms or simulation software tools to generate fault inclusive models5,6,7, and the development of fault-inclusive data sets8,9,10; (3) quantification of the prevalence and occurrence rates of faults in buildings11,12,13; (4) analysis of the impact of faults on system operations14,15, energy consumption16,17, equipment maintenance and operational costs18,19, occupant thermal comfort15,20,21, and indoor air quality22; (5) FDD technology application, costs, and benefits, in existing buildings1,23; (6) FDD algorithm performance testing methodologies24,25; and (7) automated fault correction26,27 and maintenance activities28 after faults are diagnosed and flagged by FDD tools.
Although building control and automation systems are able to store and export large volumes of operational data, these data are often prone to data quality issues including erroneous sensors and gaps. Consistent naming conventions are not used from one system to another, and semantic metadata to interpret the meaning and relationships between data are rarely used. A further complication is that the data reflect unknown and unlabeled presence of a wide variety of commonly occurring faults. Finally, while small collections of field data may be acquired by researchers, it is extremely difficult to amass a large-scale dataset that represents climate, HVAC system, and operational diversity. This presents tremendous barriers for innovation in FDD algorithm development, and performance evaluation.
Extending the body of work focused on FDD algorithm testing methods and test datasets, this paper documents a significant expansion of the HVAC fault dataset presented in9. The expansion incorporates five new HVAC systems and configurations, an increased number of fault cases, and more extensive time spans for each fault-intensity combination, (in most cases reaching a full 365 days). The data were produced using simulation tools, laboratory experimental facilities, and field tests. Additionally, a semantic model for each system has been developed according to the Brick schema29 for improved usability and conformance with today’s commonly used building industry metadata schema.
The expanded dataset documented in this article includes seven common HVAC systems: the single duct air handling unit (AHU) system, the packaged rooftop unit (RTU), the dual duct AHU system, the fan coil unit (FCU) system, the variable air volume fan power unit (FPU), the boiler plant, and the chiller plant. 257 fault cases are represented, spanning sensor-related faults, actuator-related faults, control faults (e.g., controller PID parameter settings), and component faults (e.g., cooling coil foiling fault). In total, that dataset comprises 8 billion data samples, and represents the largest known ground truth-verified data for HVAC faults. As noted in the 2020 publication9 FDD researchers and developers can use the data to:
Develop, evaluate, and compare performance across FDD algorithms;
Identify performance gaps to focus future development efforts and resource investment;
Develop an understanding of how FDD technology overall is improving over time; and
Enable a better understanding of HVAC system performance under faulted and fault-free operation conditions for educational purposes.
Prior work such as ASHRAE research projects RP-1312 and RP-1043, and National Institute of Standards and Technology (NIST) 10D243 project, represent early contributions of operational HVAC fault data. This research advances those early efforts by increasing the number and type of HVAC systems that are represented, by increasing the duration of fault-free and faulted operational span (one year in most cases), and by increasing the number and type of faults that are represented. This will significantly increase the usability of the dataset for FDD algorithm development and performance evaluation.
The newly expanded dataset contains experimental and simulated data across the seven HVAC systems types and configurations that are represented - the majority being simulated. Diverse facilities and simulation tools were used to create the data, and methods to impose the faults were created for each fault, given the specific HVAC system of focus, the control sequences that defined its operation. These facilities and tools, HVAC system details, and fault methods are described in the following, as is the metadata schema that was applied to the data. Provision of the metadata enables ease of interpretation of the data, and supports users of the dataset who wish to employ more automated procedures to interface FDD algorithm instances with the data.
The simulated datasets were created using HVACSIM+ and an EnergyPlus-Modelica co-simulation. HVACSIM + was developed by the US NIST30, the Modelica Buildings Library31 is developed by the Lawrence Berkeley National Laboratory, and EnergyPlus32 is developed by several contributors through funding from the US Department of Energy. Described with respect to other modeling tools in33, HVACSIM+, Modelica, and EnergyPlus are non-proprietary tools to model the behavior of building HVAC systems using physics-based approaches. In addition, Modelon’s air conditioning library was used to model the refrigerant side faults in the RTU system34. This library provides ready-to-use refrigeration cycle templates and a wide range of components to create a variety of air conditioning system configurations.
Four experimental research facilities were used to create data and to develop and validate simulation models:
FLEXLAB located at the Lawrence Berkeley National Laboratory in Berkeley, California, for the generation of the single-zone CAV data set and the variable-air-volume (VAV) AHU data set9.
The Flexible Research Platform (FRP) located at the Oak Ridge National Laboratory in Oak Ridge Tennessee, for the generation of RTU data sets9.
The Energy Resource Station facility was previously located at the Iowa Energy Center in Ames City, Iowa, for the development and validation of DD-AHU, FCU and FPU simulation models, and for creation of multi-zone VAV AHU data35.
The RTU facility is located in the Thermal Technology Facility (TTF) at the National Renewable Energy Laboratory in Golden, Colorado, for the validation of the RTU simulation model. NREL’s TTL is a flexible multipurpose laboratory that enables detailed evaluation and development of building and thermal energy systems. The TTF research space reaches 11,000 sq.ft. Two RTUs—a 5-ton/SEER 17 (RTU 1) and a 6-ton/IEER 23 (RTU 2) are installed in the TTL to develop comprehensive performance maps suitable for use with whole-building energy simulation computer programs. The SEER 17 contained a two-stage scroll compressor with R-410A, single-speed condenser fan, direct-drive variable-supply air fan with a high-efficiency motor, low leak dampers, hot gas reheat humidity control, and an economizer. The IEER 23 contained a variable-speed direct-drive compressor, variable-speed fans, and control logic that maintained the compressor and thermal expansion valve (TXV) within their performance limitations36.
Field data representing faulted and un-faulted rooftop unit operation is also included in the dataset. This data was collected from two RTUs, one in a restaurant building in Milford, CT and another one in a distribution center building in Colchester, CT. Table 1 summarizes these sites and the RTUs.
The configurations and sequences for each system in the data set are comprehensively documented for users of the data in an inventory file. This information is often needed to specify controls-specific parameters in fault detection and diagnostic algorithms. To illustrate the form and content of this information, two examples are presented - the fan coil unit system, and the boiler plant.
Figure 1 contains the schematic representation of the fan coil unit (FCU) system.
Schematic diagram of the FCU.
The FCU is scheduled for automatic operation on a time of day basis for occupied and unoccupied mode.
Occupied mode (Monday – Friday 6:00AM–17:59PM)
During these hours, the system is in Operate Mode. Five control sequences - control, outdoor air damper control, cooling coil valve control, heating coil valve control sequence, and zone temperature setpoints - were set during the simulation.
3-speed fan with “Automatic On/Off” (Auto) mode: the fan on/off and speed change is based on the cooling proportional-integral-derivative (PID) output and heating PID output. The 10% dead band is given at each speed switchover level.
Low speed condition: the PID outputs (the cooling/heating coil valve position) are higher than 0% and lower than 40%;
Medium speed condition: the PID outputs (the cooling/heating coil valve position) are > = 40% and <80%;
High speed condition: the PID outputs (the cooling/heating coil valve position) are > = 80% and < 100%;
Off: no heating or cooling demand.
The OA damper maintains a minimum damper position at 30%.
Cooling coil valve control sequence
The PID control is used to adjust the cooling coil valve position. The setpoint dead band is 1 °F. If the actual room temperature is beyond 1 °F of the cooling setpoint, the FCU is in the “cooling” mode, and the cooling coil valve PID loop is enabled and the cooling valve position will be controlled by the cooling coil valve controller PID output. When the room temperature falls below 1 °F compared to the cooling setpoint, the cooling PID is disabled and the valve fully closed.
Heating coil valve control sequence
The PID control is used to adjust the heating coil valve position. The setpoint dead band is 1 °F. If the actual room temperature is beyond 1 °F of the heating setpoint, the FCU is in the “heating” mode, and the heating coil valve PID loop is enabled and the heating valve position will be controlled by the heating coil valve controller PID output. When the room temperature falls below 1 °F compared to the heating setpoint, the heating PID is disabled and the valve fully closed.
The shutdown mode is only triggered by the low temperature protection described below. Under the shutdown mode, the fan is constantly off, and the OA damper is fully closed.
During the simulation, when the mixed air temperature is below 35 °F and persists for 300 seconds, the FCU system will switch to the shutdown mode to prevent freezing the coil. The shutdown mode will last until the end of the day. The system will be turned back to normal operation at the beginning of the next day.
During these hours, the system is in Setback Mode. The operation is similar to the operation mode except two additional settings as:
Outdoor air damper: The OA damper is fully closed
Figure 2 illustrates the configuration of the boiler plant system. This system has two identical boilers and two hot water pumps and provides hot water to heating coils in the air-side system.
Schematic of the studied boiler plant system.
The boiler plant system is controlled by two supervisory controllers and two local controllers (Table 2). One supervisory controller determines the number of the operating boilers using a state machine and the calculated heat load, as shown in Fig. 3. The heating load is calculated from:
where \({\mathop{v}\limits^{^\circ }}_{hw}\) is the volumetric flow rate of the hot water, \({T}_{hw}^{ent}\) and \({T}_{hw}^{lea}\) are the temperature of the hot water entering and leaving the boiler plant system, respectively. The other supervisory controller determines the number of operating hot water pumps, as shown in Fig. 4.
Staging control of boilers (ξ = 0.95 and waiting time: 30 min).
Staging control of hot water pumps in the boiler plant system (waiting time: 30 min).
Tables 3–10 summarize fault profiles and how each fault was imposed for each of the systems and fault scenarios. For the simulated datasets, each fault type and intensity were imposed for a full calendar year of operation - the exception being the simulated RTU dataset that covered a 100-day cooling season. For the experimental and field test datasets, fault type-intensity combinations were captured for one to 183 days of operation.
The RTU dataset that was acquired from field measurements reflected a naturally occurring compressor staging fault and a refrigerant undercharging fault.
The Brick schema29 offers classes and subclasses, of which the equipment class was used to designate the HVAC system components represented in the fault dataset. Similarly, the point subclass was used to design sensor measurement and control system data points. In addition, the schema offers ‘relationships’, of which hasPart, hasPoint, and feeds, are relevant to describing the fault dataset. Figure 5 illustrates the 5-step process that was used to generate the Brick models for each HVAC system in the dataset. Among them, Step 4 is automated while the other steps are performed manually.
Flow of the Brick Schema model development.
The schematic representations for each system were reviewed to identify the major components for the overall system, to develop compositional (“hasPart”) relationships. For each major component, we identify all of the associated sensor/control points to develop “hasPoint” relationships. Lastly, we identify the order in which the given media (air, water, etc.) flow through the system to develop sequential (“feeds”) relationships between different equipment.
After identifying the components and sensor/control points of the system in Step 1, we indicate which equipment has which components (“hasPart”), which equipment or component has which sensor and control data points (“hasPoint”), and which equipment feeds into another equipment (“feeds”).
All equipment, components, and sensor/control data points in the hierarchical diagram are mapped to a Brick schema class and tabulated. The equipment and the sub components are mapped to a subclass of the Brick “equipment” class (e.g., chiller, AHU, and RTU) and the sensors and the control points will be assigned a type subclass of Brick “point”.
For each row (i.e., each component), we designate the relevant relationships, other components it connected to and these components. This way, we are able to incorporate all the components, their types and how they are related to other components.
The tables generated in Step 3 are exported as CSV files and imported to a Python script that generates a Brick model in the form of a machine-readable .ttl file. The script iterates through each row of the table, assigning all components and points to a specific instantiation of a Brick class and corresponding relationships. The .ttl file can be accessed by an FDD algorithm (or other applications), enabling more efficient and standardized retrieval of system metadata using SPARQL queries. This streamlines the interpretation of data semantics within the FDD or other applications.
The generated Brick model is verified by visualizing it and comparing it to the hierarchical diagram in Step 2. We used Brick Studio for the visualization and ensured that all the components in the data sets were present and the relationships between them were labeled correctly.
The data are stored on figshare37 and on an LBNL website10. The description for the expanded seven data sets can be found in Table 11. For each system, the FDD data are stored in individual comma separated value (CSV) files, and each file contains one fault type under one fault intensity. The data are stored at the 1-minute interval rate to reflect system operations. The 1-minute interval rate can be re-sampled to a 5-minute interval and a 15-minute interval, which are also commonly used in the existing building automation system (BAS). Time stamps are in the first column of each file, and presented in the format of “yyyymmdd hh:mm”.
Each system dataset is accompanied with a .ttl Brick model and also a data ‘inventory’ file that describes the key information necessary to understand the content and scope of each data set, including:
An overview of the data set, who created it, and whether it was generated through simulation or physical experiment
Model or experimental facility description
System type and physical configuration diagram
The unit for each measurement
The basic data points which existing BASs use are labeled
Input scenarios for faulted and fault-free conditions represented in the data
Granderson et al.9 documented that the validity of the dataset can be assessed according to three dimensions: (1) accuracy of the sensors and measurement infrastructure in the experimental facilities that were used; (2) accuracy of the simulation models that were used; and (3) accuracy of the ground truth labels that indicate the presence and severity of the faults, presence or absence of faults and their severity9.
Granderson et al. describes the measurement calibration process at the FLEXLAB, FRP, and Iowa Energy Center facilities9.
Granderson et al. described the simulation model validation for the EnergyPlus-Modelica models9, and both Granderson et al. and Wen et al. describe model validation of the HVACSIM + models9,35. These publications describe a host of methods including empirical validation, experimental calibration, comparative testing (vs other tools), and analytical verification (with respect to exact solutions).
For the sake of brevity, the reader is referred to these prior publications for details on facility measurement and simulation model accuracy.
Granderson et al.9 describes a ground truth validation process that applies functional testing and engineering logic9. Functional testing verifies that system operation is consistent with the designed control sequences, and reflective of fault-free operational behavior. Engineering logic and the specified control sequence are combined to confirm that the data trends do indeed reflect the behaviors of the fault free and faulted scenarios.
Figure 6 provides a few examples for the fan coil unit system. First the data trends are inspected to confirm that the system is operated according to the defined schedule of occupied hours corresponding to 6:00–17:59, and to the defined setpoints specified in the sequence (as shown in section System configurations and control sequences). This is verified in the profile of the cooling setpoint and heating setpoint trends, which respectively modulate from 85 °F to 72 °F, and from 55 °F to 68 °F, and back at the 6:00 and 17:59 timestamps. Next, the data trends are inspected to verify that the modeled PID parameters for the cooling valve controller are configured to output proper control signals. This is confirmed through smooth trend and absence of any significant oscillations in the plotted signal for the cooling coil valve command. Finally, inspection of the zone temperature trend confirms that the control objective, i.e., a cooling setpoint of 72 °F, was maintained throughout the occupied period of operation.
Example of FCU fault free operational data (July 17).
Following verification of the fault-free operational state, additional tests were conducted for each of the faulted scenarios. These tests considered (a) whether the imposed fault condition was correctly reflected in the data, and (b) whether the anticipated symptoms of the fault were reflected in other operational trends.
Figure 7 illustrates these two types of tests for the FCU system fault - zone air temperature sensor bias of +2 °C (3.6 °F). The biased condition is confirmed by comparing the 2 °C offset between the data trend from the ‘spoofed’ faulted model output point (solid line), and the unaltered output point (dashed line). This is clearly discernible and annotated in the righthand portion of the plot. The symptoms of this bias are observed in comparing the cooling coil valve position in the faulted case (the black solid line) to that from the unfaulted case (dashed black line). The position in the faulted case is significantly higher because the controller was attempting to provide an increased amount of cooling commensurate with the erroneously high zone air temperature reading.
Example of FCU fault present operational data (zone air temperature sensor bias +2 °C (3.6 °F), July 17).
Figure 8 illustrates another FCU system fault - cooling coil valve stuck at 20%, imposed during the cooling season. Here, the faulted condition is confirmed by observing that the valve position signal (black solid line) is fixed at 0.2, while the valve command signal (black dashed line) is adjusted. The symptom of this fault is that the zone temperature (purple solid line) significantly exceeded the 72 °F cooling setpoint during the occupied hours even though the cooling coil valve control signal (black dashed line) reached a value of 1 (i.e., 100% position) in the controller’s attempt to provide maximum cooling.
Example of FCU fault present operational data (cooling coil valve stuck fault at 20% position, July 17).
Similar verification tests steps were performed for each fault type at each intensity level in experimental data sets and simulation data sets. For the simulated data sets that spanned a full year of operation, a sample of at least three days was selected for inspection from each of three operational seasons - summer/cooling season, winter/heating season and a transitional/swing season. This sampling enabled validation of the data and faulted system behaviors under different weather conditions and operational modes38.
A complete inventory of the data was developed to support users in interpreting the content and form of the data, and the corresponding HVAC systems, controls, and faults. The data itself comprise time series that can be analyzed with whatever software tools the user elects to implement. The data are provided at 1-minute intervals, and can be resampled as needed to fit the needs of specific applications.
The Modelica Buildings Library and EnergyPlus are freely available for download39,40. EnergyPlus runs on Windows, Mac OSX, and Linux operating systems. A Windows or Linux-based computer and Dymola solver are required to run Modelica, and Dymola can be licensed from Modelica Buildings Library. HVACSIM + is also freely available, upon request from NIST, and has no operating system requirements. The Modelon air conditioning library that was used to model the faults in the RTU refrigerant side, was accessed from Modelon’s library suite34. The Modelica-based library is used to design, analyze and optimize air conditioning systems.
A custom Python-based script was developed to create Brick model .ttl files for each system in the dataset, following the process described in Method of Brick schema model development. The .ttl files are included in the data repository.
Lin, G., Kramer, H., Nibler, V., Crowe, E. & Granderson, J. Building Analytics Tool Deployment at Scale: Benefits, Costs, and Deployment Practices. Energies. 15(13), 4858, https://doi.org/10.3390/en15134858 (2022).
Katipamula, S. & Brambley, M. R. Methods for Fault Detection, Diagnostics, and Prognostics for Building Systems—A Review, Part I. HVAC&R Research. 11(1), 3–25, https://doi.org/10.1080/10789669.2005.10391123 (2005).
Katipamula, S. & Brambley, M. R. Methods for Fault Detection, Diagnostics, and Prognostics for Building Systems—A Review, Part II. HVAC&R Research. 11(2), 169–187, https://doi.org/10.1080/10789669.2005.10391133 (2005).
Kim, W. & Katipamula, S. A review of fault detection and diagnostics methods for building systems. Science and Technology for the Built Environment. 24(1), 3–21, https://doi.org/10.1080/23744731.2017.1318008 (2018).
Li, Y. & O’Neill, Z. A critical review of fault modeling of HVAC systems in buildings. Build Simul. 11(5), 953–975, https://doi.org/10.1007/s12273-018-0458-4 (2018).
Pourarian, S. et al. A tool for evaluating fault detection and diagnostic methods for fan coil units. Energy and Buildings. 136, 151–160 (2017).
Zhang, R. & Hong, T. Modeling of HVAC operational faults in building performance simulation. Applied Energy. 202, 178–188, https://doi.org/10.1016/j.apenergy.2017.05.153 (2017).
Comstock, M., Braun, J. Development of Analysis Tools for the Evaluation of Fault Detection and Diagnostics in Chillers, ASHRAE Research Project RP-1043. Ray W. Herrick Laboratory Purdue University; (1999).
Granderson, J., Lin, G., Harding, A., Im, P. & Chen, Y. Building fault detection data to aid diagnostic algorithm creation and performance testing. Sci Data. 7(1), 65, https://doi.org/10.1038/s41597-020-0398-6 (2020).
Article PubMed PubMed Central Google Scholar
Granderson, J., Lin, G., Chen, Y., Casillas, A. LBNL Fault Detection and Diagnostics Datasets. DOE Open Energy Data Initiative (OEDI); Lawrence Berkeley National Lab. (LBNL), Berkeley, CA (United States); https://data.openei.org/submissions/5763, https://faultdetection.lbl.gov/, https://doi.org/10.25984/1881324 (2022).
Comstock, M. C., Braun, J. E. & Groll, E. A. A survey of common faults for chillers. ASHRAE Transactions. 108, 819 (2002).
Crowe, E. et al. What We Learned From Analyzing 15 Million Rows of Commercial Buildings’ HVAC Fault Data. In: 2022 Summer Study on Energy Efficiency in Buildings Proceedings. (2022).
Kim, J. et al. Research challenges and directions in HVAC fault prevalence. Science and Technology for the Built Environment. 27(5), 624–640, https://doi.org/10.1080/23744731.2021.1898243 (2021).
Chen, Y., Lin, G., Chen, Z., Wen, J. & Granderson, J. A simulation-based evaluation of fan coil unit fault effects. Energy and Buildings. 263, 112041, https://doi.org/10.1016/j.enbuild.2022.112041 (2022).
Li, Y. & O’Neill, Z. An innovative fault impact analysis framework for enhancing building operations. Energy and Buildings. 199, 311–331 (2019).
Roth, K., Westphalen, D., Llana, P., Feng, M. The Energy Impact of Faults in U.S. Commercial Buildings. In: International Refrigeration and Air Conditioning Conference. Purdue University; 2004.
Wang, S. & Xiao, F. AHU sensor fault diagnosis using principal component analysis method. Energy and Buildings. 36(2), 147–160, https://doi.org/10.1016/j.enbuild.2003.10.002 (2004).
Breuker, M. S. & Braun, J. E. Common faults and their impacts for rooftop air conditioners. HVAC&R Research. 4(3), 303–318, https://doi.org/10.1080/10789669.1998.10391406 (1998).
Salah, M., Osman, H. & Hosny, O. Performance-Based Reliability-Centered Maintenance Planning for Hospital Facilities. Journal of Performance of Constructed Facilities. 32(1), 04017113, https://doi.org/10.1061/(ASCE)CF.1943-5509.0001112 (2018).
Gunay, B. et al. Energy and comfort performance benefits of early detection of building sensor and actuator faults. Building Services Engineering Research and Technology. 39(6), 652–666 (2018).
Chen, Y., Chen, Z., Lin, G., Wen, J., Granderson, J. A Simulation-Based Method to Analyze Fan Coil Unit Fault Impacts. In: ASHRAE Transactions. Vol 128. ASHRAE; 210. https://www.ashrae.org/technical-resources/ashrae-transactions (2023).
Ginestet, S., Marchio, D. & Morisot, O. Evaluation of faults impacts on energy consumption and indoor air quality on an air handling unit. Energy and Buildings. 40(1), 51–57, https://doi.org/10.1016/j.enbuild.2007.01.012 (2008).
Granderson, J., G.R. et al. Commercial Fault Detection and Diagnostics Tools: What They Offer, How They Differ, and What’s Still Needed. Lawrence Berkeley National Lab.(LBNL), Berkeley, CA (United States); 2018. https://escholarship.org/uc/item/4j72k57p Accessed November 23, (2021).
Frank, S. et al. A performance evaluation framework for building fault detection and diagnosis algorithms. Energy and Buildings. 192, 84–92, https://doi.org/10.1016/j.enbuild.2019.03.024 (2019).
Yuill, D. P. & Braun, J. E. Evaluating the performance of fault detection and diagnostics protocols applied to air-cooled unitary air-conditioning equipment. HVAC&R Research. 19(7), 882–891, https://doi.org/10.1080/10789669.2013.808135 (2013).
Lin, G., Pritoni, M., Chen, Y. & Granderson, J. Development and Implementation of Fault-Correction Algorithms in Fault Detection and Diagnostics Tools. Energies. 13(10), 2598, https://doi.org/10.3390/en13102598 (2020).
Pritoni, M. et al. From fault-detection to automated fault correction: A field study. Building and Environment. 214, 108900, https://doi.org/10.1016/j.buildenv.2022.108900 (2022).
Eskandari, N. & Noorzai, E. Offering a preventive solution to defects in commercial building facility system using BIM. Facilities. 39(13/14), 859–887, https://doi.org/10.1108/F-04-2020-0037 (2021).
Balaji, B. et al. Brick: Metadata schema for portable smart building applications. Applied Energy. 226, 1273–1292, https://doi.org/10.1016/j.apenergy.2018.02.091 (2018).
Clark, D. R., May W. B. HVACSIM+ Building Systems and Equipment Simulation Program - User’s Guide. National Bureau of Standards, Building Equipment Division; 1985. https://www.osti.gov/biblio/6127383 Accessed November 20, (2021).
Wetter, M., Zuo, W., Nouidui, T. S. & Pang, X. Modelica Buildings library. Journal of Building Performance Simulation. 7(4), 253–270, https://doi.org/10.1080/19401493.2013.765506 (2014).
Crawley, D. B. et al. EnergyPlus: creating a new-generation building energy simulation program. Energy and Buildings. 33(4), 319–331, https://doi.org/10.1016/S0378-7788(00)00114-6 (2001).
Pourarian, S., Kearsley, A., Wen, J. & Pertzborn, A. Efficient and robust optimization for building energy simulation. Energy and Buildings. 122, 53–62, https://doi.org/10.1016/j.enbuild.2016.04.019 (2016).
Article PubMed PubMed Central Google Scholar
Modelon. Air Conditioning Library. Modelon. Published 2020. https://modelon.com/library/air-conditioning-library/Accessed October 31, (2022).
Wen, J., Pourarian, S., Yang, X., Li, X. NIST 10D243 Tools for Evaluating Fault Detection and Diagnostic Methods for HVAC Secondary Systems of a Net Zero Building. The US National Institute of Standard & Technology; 2015. https://www.researchgate.net/profile/Jin-Wen-12/publication/337946061_NIST10D243_FinalReport_-Tools_for_Evaluating_Fault_Detection_and_Diagnostic_Methods_for_HVAC_Secondary_Systems_of_a_Net_Zero_Building/links/5df73f11299bf10bc35f1163/NIST10D243-FinalReport-Tools-for-Evaluating-Fault-Detection-and-Diagnostic-Methods-for-HVAC-Secondary-Systems-of-a-Net-Zero-Building.pdf Accessed January 25, (2021).
Wheeler, G., Kozubal, E., Judkoff, R. Experimental Design and Laboratory Characterization of a Medium- and High-Efficiency Rooftop Unit for use in Building Energy Simulations. International Refrigeration and Air Conditioning Conference. https://docs.lib.purdue.edu/iracc/2057 Published online January 1, (2018).
Granderson, J. LBNL FDD Data Sets. figshare https://doi.org/10.6084/m9.figshare.c.6486349.v1 (2023).
Casillas, A., Lin, G., Granderson, J. Curation of Ground-Truth Validated Benchmarking Datasets for Fault Detection Diagnostics Tools. In: 2020 ACEEE Summer Study on Energy Efficiency in Buildings.; 2020. https://escholarship.org/uc/item/9cz491j6 Accessed November 20, (2021).
Lawrence Berkeley National Lab. Modelica Buildings Library. Published December 6, 2022. https://simulationresearch.lbl.gov/modelica.html Accessed January 1, (2023).
Department of Energy, U.S. EnergyPlus. Published 2022. https://energyplus.net/ Accessed January 1 (2023).
This work was supported by the Assistant Secretary for Energy Efficiency and Renewable Energy, Building Technologies Office, of the U.S. Department of Energy under Contract No. DE-AC02-05CH11231. The authors wish to acknowledge Ravi Gorthala, University of New Haven, for his contribution of in-situ RTU field data to the dataset. We would like to thank Dr. Sungkyun Jung, Oak Ridge National Laboratory, for his contribution of the simulation RTU data to the dataset. In addition, we thank Brian Walker and Erika Gupta (previously) of the Building Technologies Office for their generous support.
Lawrence Berkeley National Laboratory, Berkeley, USA
Jessica Granderson, Guanjing Lin, Yimin Chen & Armando Casillas
Jin Wen & Zhelun Chen
Oak Ridge National Laboratory, Oak Ridge, USA
Piljae Im & Sen Huang
National Renewable Energy Laboratory, Golden, USA
You can also search for this author in PubMed Google Scholar
You can also search for this author in PubMed Google Scholar
You can also search for this author in PubMed Google Scholar
You can also search for this author in PubMed Google Scholar
You can also search for this author in PubMed Google Scholar
You can also search for this author in PubMed Google Scholar
You can also search for this author in PubMed Google Scholar
You can also search for this author in PubMed Google Scholar
You can also search for this author in PubMed Google Scholar
Jessica Granderson led the overall research effort; wrote the original manuscript; reviewed and edited the manuscript. Yimin Chen wrote the original manuscript; curated and synthesized the datasets; synthesized the datasets; and documented the data. Guanjing Lin synthesized the datasets; and led the technical validation and documentation of the data; reviewed the manuscript. Armando Cassilas curated and synthesized the datasets; and documented the data. Jin Wen curated and synthesized the DDAHU, FCU and FPU datasets; and documented the data. Zhelun Chen curated and synthesized the DDAHU, FCU and FPU datasets; and documented the data. Piljae Im curated and synthesized the RTU datasets. Sen Huang curated and synthesized the SDAHU, chiller plant and boiler plant datasets; and documented the data. Jiazhen Ling curated and synthesized the RTU dataset; and documented the data.
Correspondence to Jessica Granderson or Yimin Chen.
The authors declare no competing interests.
Publisher’s note Springer Nature remains neutral with regard to jurisdictional claims in published maps and institutional affiliations.
Open Access This article is licensed under a Creative Commons Attribution 4.0 International License, which permits use, sharing, adaptation, distribution and reproduction in any medium or format, as long as you give appropriate credit to the original author(s) and the source, provide a link to the Creative Commons license, and indicate if changes were made. The images or other third party material in this article are included in the article’s Creative Commons license, unless indicated otherwise in a credit line to the material. If material is not included in the article’s Creative Commons license and your intended use is not permitted by statutory regulation or exceeds the permitted use, you will need to obtain permission directly from the copyright holder. To view a copy of this license, visit http://creativecommons.org/licenses/by/4.0/.
Granderson, J., Lin, G., Chen, Y. et al. A labeled dataset for building HVAC systems operating in faulted and fault-free states. Sci Data 10, 342 (2023). https://doi.org/10.1038/s41597-023-02197-w
DOI: https://doi.org/10.1038/s41597-023-02197-w
Anyone you share the following link with will be able to read this content:
Sorry, a shareable link is not currently available for this article.
Provided by the Springer Nature SharedIt content-sharing initiative
Scientific Data (Sci Data) ISSN 2052-4463 (online)
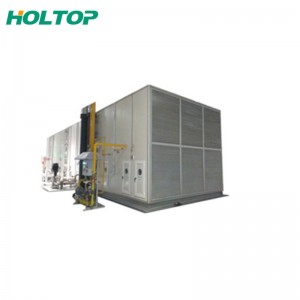
On Air Conditioner Sign up for the Nature Briefing newsletter — what matters in science, free to your inbox daily.